Data Science Course In Hyderabad
Data Science Training In Hyderabad With Placement
Upgrade your Data Science Skillset with our Data Analyst courses in Hyderabad!
Trained 15000+ Students | Course duration: 40 hours | Real-time Project Execution | Certification exam after course completion | Basic to advanced level learning |
Key Features
Course Duration : 8 Weeks
Live Projects : 1
Online Live Training
EMI Option Available
Certification & Job Assistance
24 x 7 Lifetime Support
Our Industry Expert Trainer
We are a team of 10+ Years of Industry Experienced Trainers, who conduct the training with real-time scenarios.
The Global Certified Trainers are Excellent in knowledge and highly professionals.
The Trainers follow the Project-Based Learning Method in the Interactive sessions.
Overview of Data Science Training Course in Hyderabad
Looking to build a rewarding career in data science? 3RI Technologies offers an industry-focused data science course in Hyderabad, tailored for both beginners and working professionals. Recognized among the best data science institutes in Hyderabad, we provide comprehensive data science training in Hyderabad that covers the latest tools, techniques, and trends. Our curriculum is carefully designed to match the needs of the real-world job market, making us a preferred data science training institute in Hyderabad.
Whether you are searching for a reliable data science institute Hyderabad, exploring top data science courses Hyderabad, or need data science training in Hyderabad with placement support, 3RI Technologies delivers it all. We also offer flexible learning options like data science online training in Hyderabad for remote learners. Our data science course in Hyderabad with placements ensures that you gain not just knowledge, but real career opportunities too.
Learn from one of the top-rated data science institutes in Hyderabad at an affordable data science course fee in Hyderabad. Dive into the world of analytics with a trusted data scientist institute in Hyderabad, covering everything in our updated data science course institutes in Hyderabad directory. Your future in data science Hyderabad starts here!
Data Science Course features
- Live Sessions
- Mocks, Assignments, & Tests
- Job Assistance
- 24/7 Lifetime Technical Support
- 10+ years of experience Proficient
- Real-time project experience
- Flexible Timings
Prerequisites
Basic knowledge of Python programming language, SQL, and files (MS Excel, CSV, etc.) with knowledge about algebra and geometry.
Course Duration
40 hours, i.e., 8-9 weeks approx.
Who all can apply for this course?
- Career switch Developers
- Candidates willing to start their career in Data Science or data analytics field
- Machine Learning or Hadoop background developers
- Data Analysts
- Business Analysts
Skills Required
- No Prerequisites for Data Science certification training
- Basic knowledge of SQL is advantageous
Data Science Course Syllabus
Decade Years Legacy of Excellence | Multiple Cities | Manifold Campuses | Global Career Offers
- Fundamentals of Data Science and Machine Learning
- Introduction to Data Science
- Need of Data Science
- BigData and Data Science’
- Data Science and machine learning
- Data Science Life Cycle
- Data Science Platform
- Data Science Use Cases
- Skill Required for Data Science
- Mathematics For Data Science
- Linear Algebra
- Vectors
- Matrices
- Optimization
- Theory Of optimization
- Gradients Descent
- Introduction to Statistics
- Descriptive vs. Inferential Statistics
- Types of data
- Measures of central tendency and dispersion
- Hypothesis & inferences
- Hypothesis Testing
- Confidence Interval
- Central Limit Theorem
- Probability and Probability Distributions
- Probability Theory
- Conditional Probability
- Data Distribution
- Distribution Functions
- Normal Distribution
- Binomial Distribution
- An Introduction to Python
- Why Python , its Unique Feature and where to use it?
- Python environment Setup/shell
- Installing Anaconda
- Understanding the Jupyter notebook
- Python Identifiers, Keywords
- Discussion about installed module s and packages
- Conditional Statement ,Loops and File Handling
- Python Data Types and Variable
- Condition and Loops in Python
- Decorators
- Python Modules & Packages
- Python Files and Directories manipulations
- Use various files and directory functions for OS operations
- Python Core Objects and Functions
- Built in modules (Library Functions)
- Numeric and Math’s Module
- String/List/Dictionaries/Tuple
- Complex Data structures in Python
- Python built in function
- Python user defined functions
4. Introduction to NumPy
- Array Operations
- Arrays Functions
- Array Mathematics
- Array Manipulation
- Array I/O
- Importing Files with Numpy
5. Data Manipulation with Pandas
- Data Frames
- I/O
- Selection in DFs
- Retrieving in DFs
- Applying Functions
- Reshaping the DFs – Pivot
- Combining DFs
Merge
Join - Data AlignmentÂ
6. SciPy
- Matrices Operations
- Create matrices
Inverse, Transpose, Trace,  Norms , Rank etc - Matrices Decomposition
Eigen Values & vectors
SVDs
7. MatPlotLib & Seaborn
- Basics of Plotting
- Plots Generation
- Customization
- Store Plots
8. SciKit Learn
- Basics
- Data Loading
- Train/Test Data generation
- Preprocessing
- Generate Model
- Evaluate Models
9. Descriptive Statistics
. Data understanding
- Observations, variables, and data matrices
- Types of variables
- Measures of Central Tendency
- Arithmetic Mean / Average
- Merits & Demerits of Arithmetic Mean and Mode
- Merits & Demerits of Mode and Median
- Merits & Demerits of Median Variance
10. Probability Basics
- Notation and Terminology
- Unions and Intersections
- Conditional Probability and Independence
11. Probability Distributions
- Random Variable
- Probability Distributions
- Probability Mass Function
- Parameters vs. Statistics
- Binomial Distribution
- Poisson Distribution
- Normal Distribution
- Standard Normal Distribution
- Central Limit Theorem
- Cumulative Distribution function
12. Tests of Hypothesis
- Large Sample Test
- Small Sample Test
- One Sample: Testing Population Mean
- Hypothesis in One Sample z-test
- Two Sample: Testing Population Mean
- One Sample t-test – Two Sample t-test
- Paired t-test
- Hypothesis in Paired Samples t-test
- Chi-Square test
13. Data Analysis
- Case study- Netflix
- Deep analysis on Netflix data
- Exploratory Data Analysis
- Data Exploration
- Missing Value handling
- Outliers Handling
- Feature Engineering
- Feature Selection
- Importance of Feature Selection in Machine Learning
- Filter Methods
- Wrapper Methods
- Embedded Methods
- Machine Learning: Supervised Algorithms Classification
- Introduction to Machine Learning
- Logistic Regression
- Naïve Bays Algorithm
- K-Nearest Neighbor Algorithm
- Decision Tress
- SingleTree
- Random Forest
- Support Vector Machines
- Model Ensemble
- Model Evaluation and performance
- K-Fold Cross Validation
- ROC, AUC etc…
- Hyper parameter tuning
- Regression
- classification
- Machine Learning: Regression
- Simple Linear Regression
- Multiple Linear Regression
- Decision Tree and Random Forest Regression
- Machine Learning: Unsupervised Learning Algorithms
- Similarity Measures
- Cluster Analysis and Similarity Measures
- Ensemble algorithms
- Bagging
- Boosting
- Voting
- Stacking
- K-means Clustering
- Hierarchical Clustering
- Principal Components Analysis
- Association Rules Mining & Market Basket Analysis
- Machine Learning end to end Project blueprint
- Case study on real data after each model.
- Regression predictive modeling – E-commerce
- Classification predictive modeling – Binary Classification
- Case study on Binary Classification – Bank Marketing
- Case study on Sales Forecasting and market analysis
- Widespread coverage for each Topic
- Various Approaches to Solve Data Science Problem
- Pros and Cons of Various Algorithms and approaches
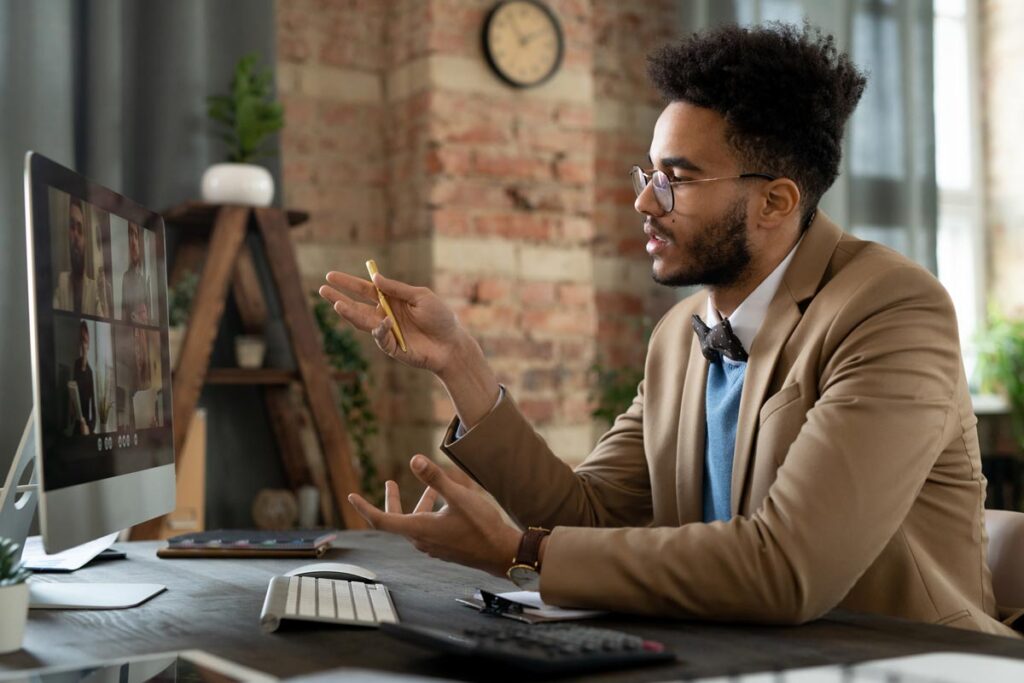
Claim your free expert counseling session today!
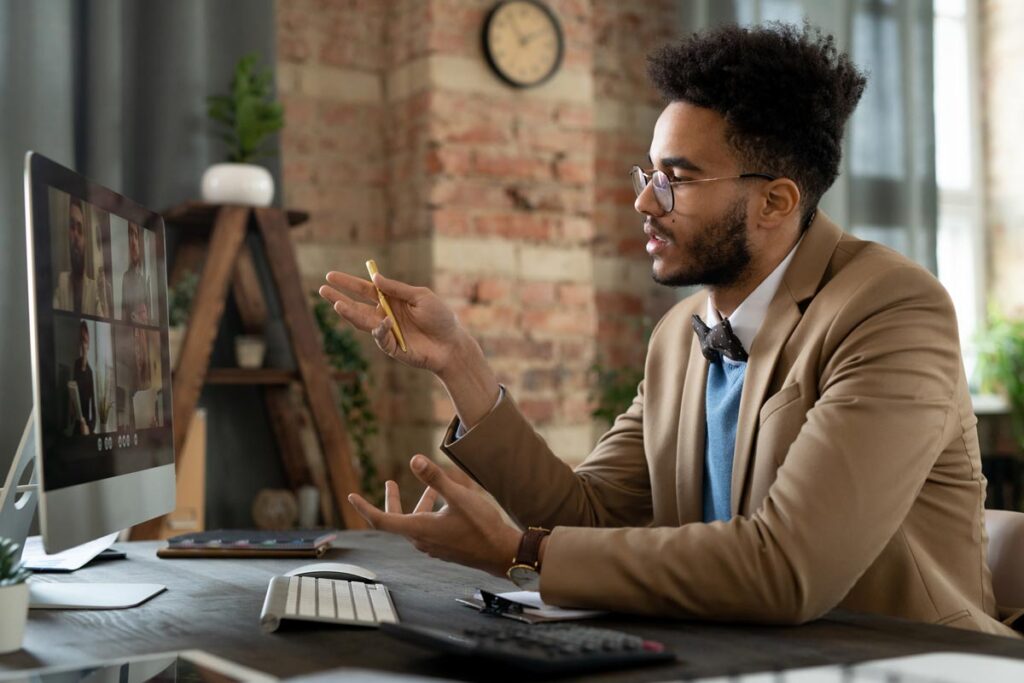
Do you want to book a FREE Demo Session?
Who can apply for the course?
- Aspiring Data Scientists who are interested in switching careers.
- Graduate/post-graduate students wishing to pursue their careers in Data Analytics/Data Science.
- Professionals who work with big data.
- Professionals from non-IT bkg, and want to establish in IT.
- Candidate who would like to restart their career after a gap.
- Machine learning is a topic of interest to professionals.
- Business analysts and those who work with data
Want an Expert Opinion?
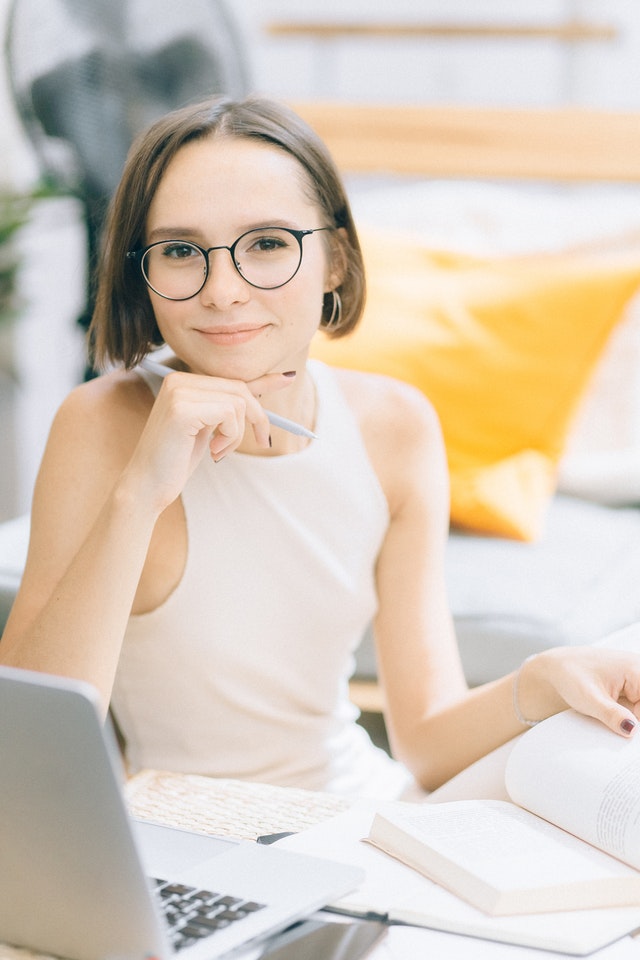
Industry Projects
Learn through real-life industry projects sponsored by top companies across industries
- Project Implementation with Real-Time Scenario.
Dedicated Industry Experts Mentors
Receive 1:1 career counselling sessions & mock interviews with hiring managers. Further your career with our 300+ hiring partners.
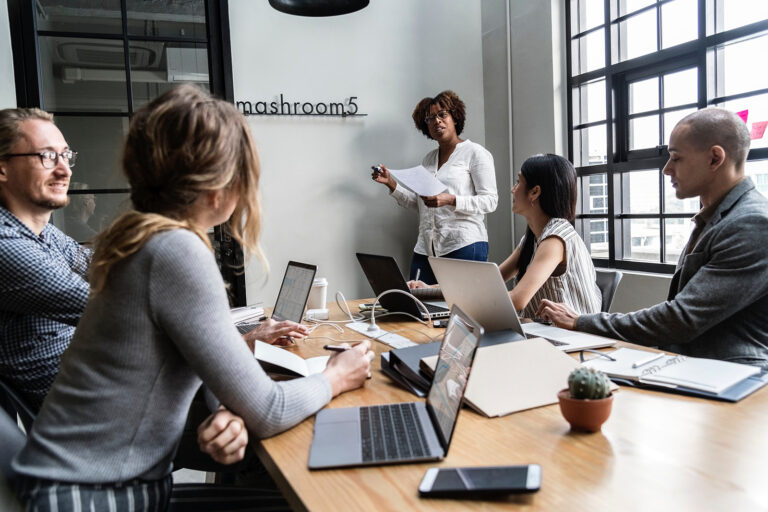
I'm Interested in This Program
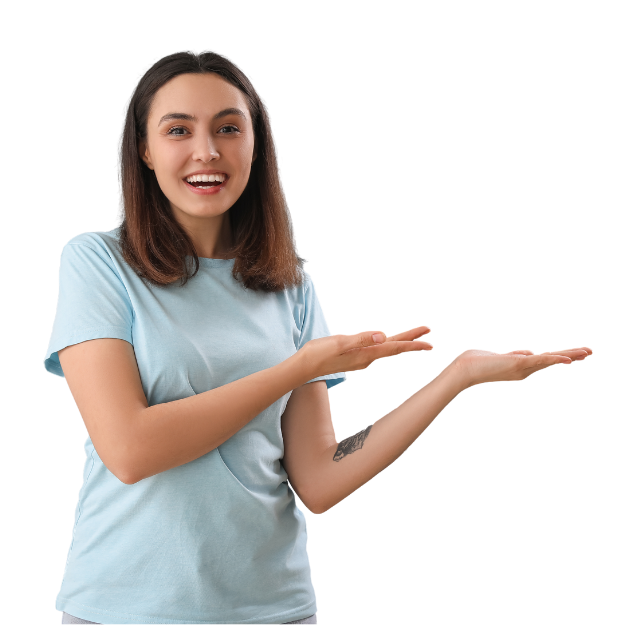
Our Clients
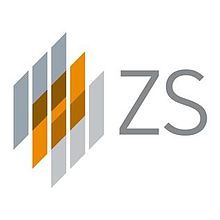
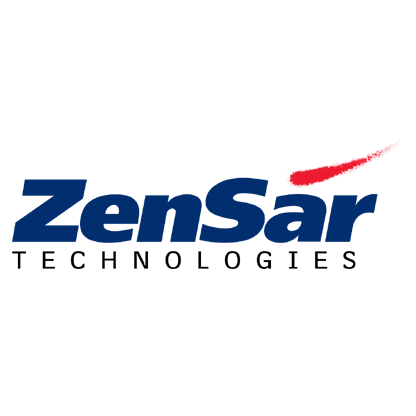
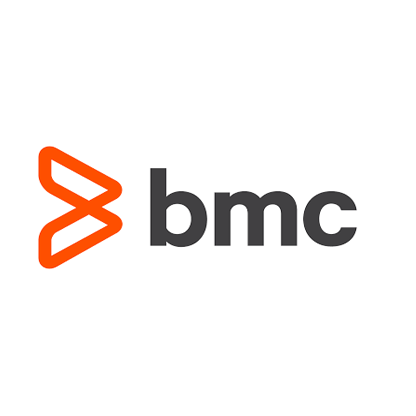
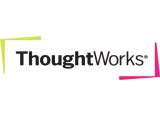
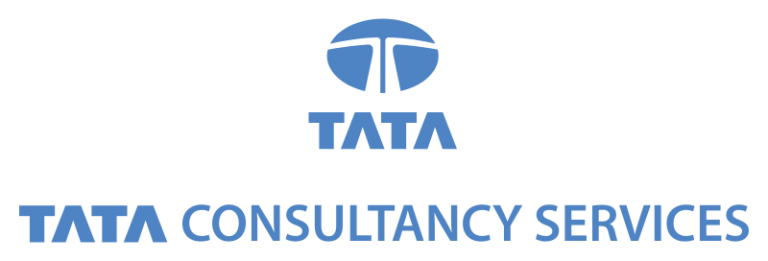
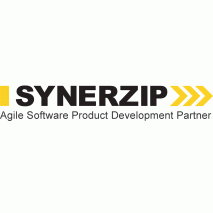
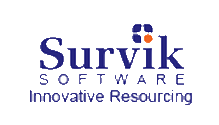
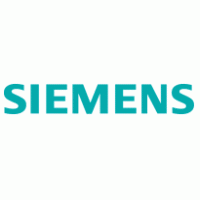
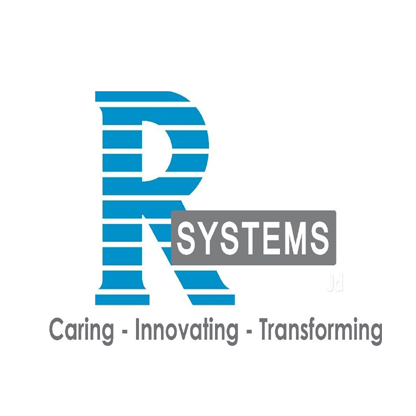
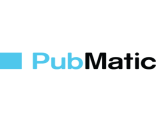
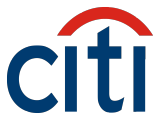
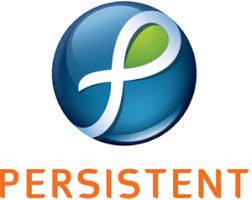
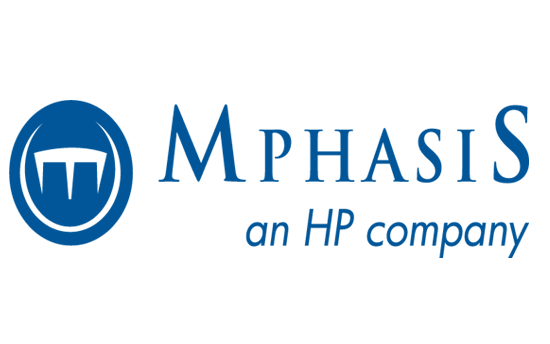
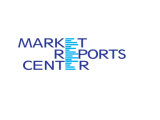
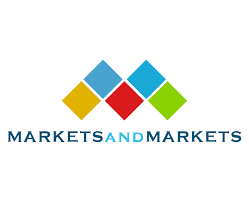
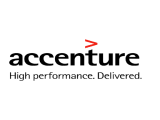
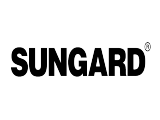
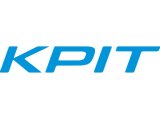
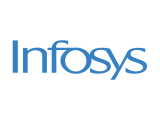
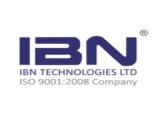
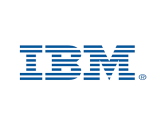
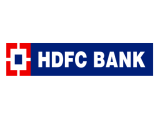
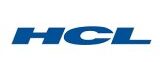
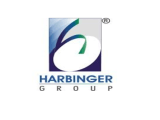
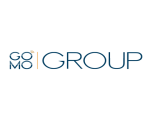
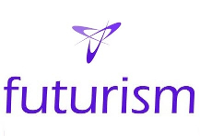
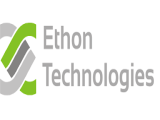
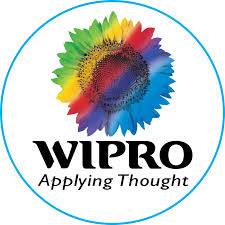
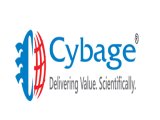
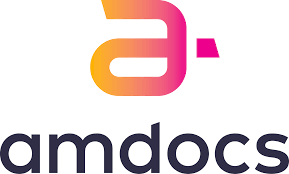
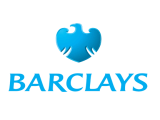
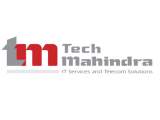
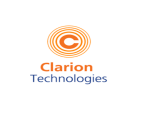
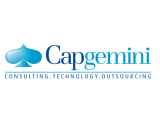
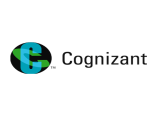
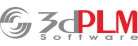
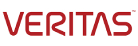
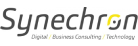
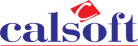
Data Science Training Testimonials
What our students talks about us. If you were student of 3RI and wants to share your thought about us, kindly mail or call us.